Fu X, Huang J, Zeng D, et al. Removing rain from single images via a deep detail network[C]//Proceedings of the IEEE Conference on Computer Vision and Pattern Recognition. 2017: 3855-3863.
1. Overview
- 当object的structure, orientation与rain streak相似时,基于low-level图像特征的de-rain方法效果很差
- ResNet通过改变映射形式简化训练过程
因此,论文提出用于de-rain的Deep Detail Network框架
- 利用lossless negative residual mapping, 缩小映射范围,简化训练过程.
(输入)rain image - (预测)rain residual = (输出)clean image

- 引入prior信息(rain high-frequency).
- 去除图片背景,得到包含object and rain streaks structures的图片,将其作为神经网络的输入,神经网络对rain structure建模.
- 此外,去除背景后的图片像素值范围缩小,且大部分像素点为0,其稀疏性能够提高效果。
- Y: gt; X: rain image; X_detail: obj and rain streak structure; |Y - X|: rain structure.

- 创建数据集.
1.1. 框架特点
- 可泛化到真实图像
- 通用性. 能用于去噪,降低JPEG artifacts
- 基于先验信息缩小映射范围(而ResNet改变映射形式)
1.2. 数据集
- 从UCID、BSD和Google image search中收集1000张clean image
- 每张图片生成14种不同streak orientation and magnitude的rain image
1.3. 相关工作
分为两类
- 基于视频
- 基于单张图片
- 基于视频的de-rain任务可以使用inter-frame information,相对于基于单张图片而言,较简单。
基于单张图片的工作有:
- Non-local mean fltering
- GMM + patch-rank prior (low-rank)
- (patch-based)
- GMM + patch-based prior
- DSC (discriminative sparse coding). Dictionary Learning
1.4. Findings
heavy rain image存在类似于haze的现象,因此,对图片进行de-haze预处理能够提高效果。

2. Deep Detail Network

2.1. Direct Network

- 神经网络输入(X): rain image
- 神经网络输出>: clean image
- 出现color shift现象.
frobenius范数
平方-求和-开方

2.2. Negative Residual Mapping

- 神经网络输入(X): rain image
- 神经网络输出: rain structure
- 模型输出: clean image = rain image - rain structure
- gt: clean image
通过缩小输出映射范围,减小solution space. 无法完全de-rain.
2.3. Deep Detail Network

- 神经网络输入(X_detail): obj + rain structure image
- 神经网络输出: rain structure
- 模型输出: clean image = rain image - rain structure
- gt: clean image
输入具有稀疏性 + 输出缩小映射范围.

2.3.1. Decompose
使用guided filtering(实验中,半径设为15)作为low-pass filter,将图片分为
- Base layer
- Detail layer
Detail layer包含图片中object and rain streak structure信息.

2.4. 网络结构

3. Experiments
实验与两个算法进行对比
- [25] DSC
- [24] GMM + Patch-rank prior
评价指标SSIM
3.1. 合成数据


3.2. 真实数据

3.3. 测试时间
[24] [25]算法为CPU运行时间。
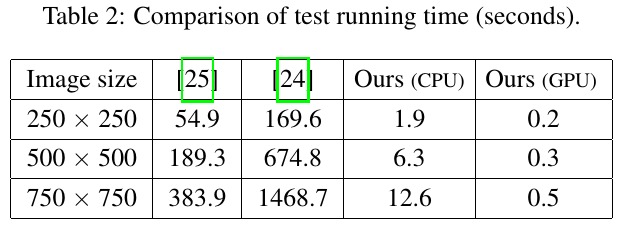
3.4. 收敛比较
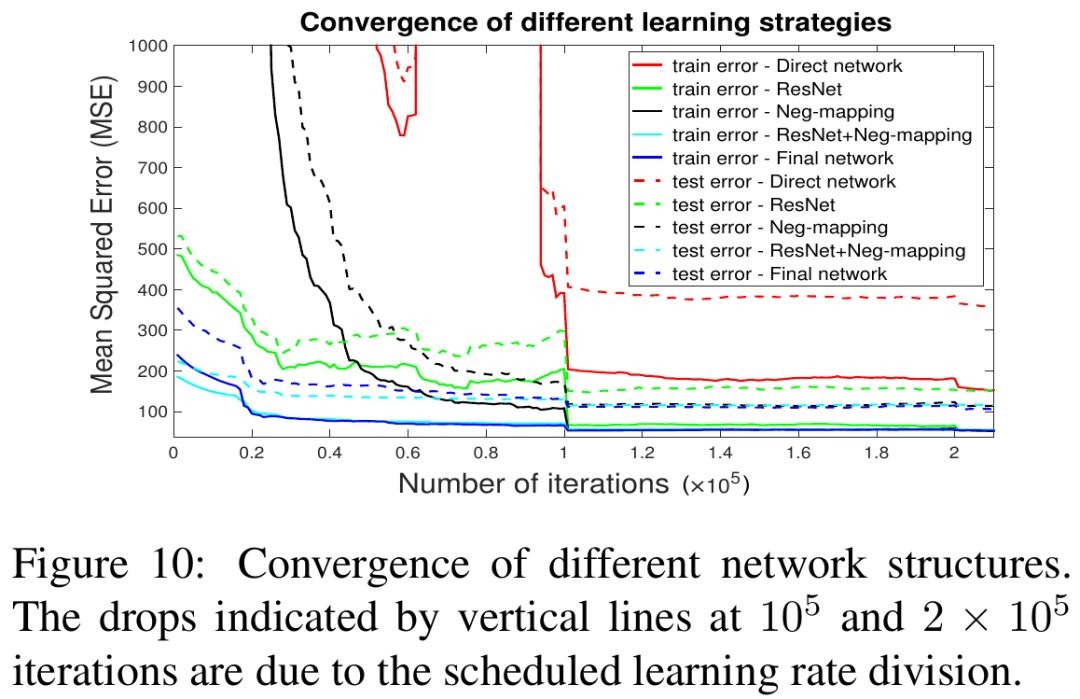
3.5. 网络depth vs width



3.6. 与深度学习算法(ICCV 2013)比较

3.7. 扩展任务(通用性)
